ServiceNow Incident Status Synchronization
by Mahdi KarabibenYou can now automatically update your Sifflet incidents based on the progress tracked in ServiceNow. This feature synchronizes status changes from a linked ServiceNow incident directly to its corresponding Sifflet incident.
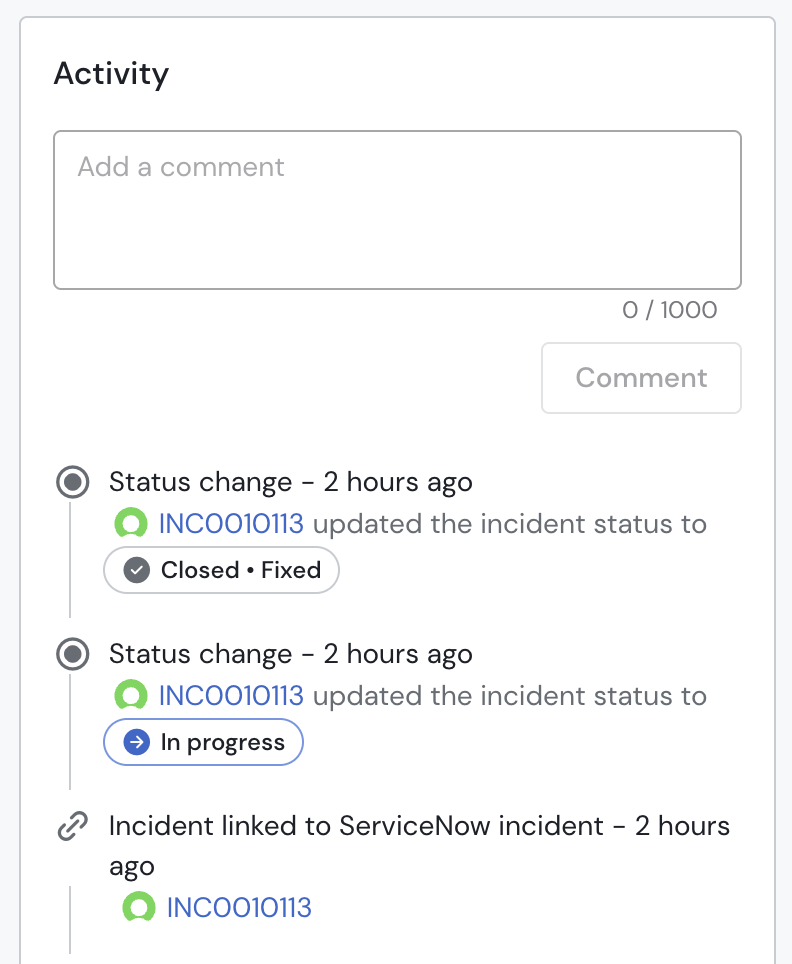
ServiceNow incident status updates in the Sifflet incident timeline.
- How it works: When a linked ServiceNow incident's state transitions to specific 'In Progress' or 'Resolved/Closed/Cancelled' values, the corresponding Sifflet incident's status will automatically update to 'In progress' or 'Closed - Fixed' based on a mapping that you provide.
- Configuration required: To enable this synchronization, activate the "Enable status changes sync from ServiceNow to Sifflet" toggle within the Sifflet ServiceNow integration settings. Additionally, a specific Business Rule needs to be configured in your ServiceNow instance using the script provided in our documentation.
- Benefit: Ensures that incident progress and resolution tracked in ServiceNow are accurately and automatically reflected within Sifflet, improving visibility for users monitoring data quality incidents in Sifflet and reducing the need for manual status updates.
Learn more about configuring ServiceNow Incident Status Synchronization via the dedicated docs page.
App version: v472